Aria: AI-Powered Music Discovery Tailored to Users' Preferences
Amazon Music Challenge
Problem
Users' moods and music tastes are dynamic but the conventional personalized playlists do not often consider that. Also, users sometimes want to discover music that they don't usually listen to.
Solution
Aria = Sister of Alexa. Design a feature that utilizes AI to create personalized music playlists that deeply adjust to the user's preferences.
Project Type
Amazon Music Challenge
Duration
February 2024 to March 2024 (1 month)
Team
Yuri Minami (designer) + 2 designers
My Role
Product Design. We collaborated in each phase. After the project ends, I made improvements individually based on our program mentor's feedbacks.
This project was the student competition held by Amazon Music, with the following prompt. "Given the current state of Amazon Music, how might AI enhance a customer’s experience with music?"
A study showed that GenZ and Millennials spend more time on music streaming services than older generation (Data from musical pursuits). Also, the data revealed 81% of US internet users aged 18 to 34 have a paid Amazon Prime membership (Data from Search Logistics). Since Prime users have access to Amazon Music, we assume that addressing young users' problems and needs could increase their usage of Amazon Music. Thats' why we focused on young users in this project.
We conducted user interviews with 12 people from an age group of 20-35 to ask about their music listening experiences. We created an affinity diagram to organize our interview results. We also conducted user surveys to learn about user’s preferences for content discovery and AI usage further. We collected responses from 32 people. Based on them, we made a persona.
User Research Findings
3.37/5
AI music recommendation accuracy satisfaction
Average rate of satisfaction with AI music recommendation. There is still a room for improvement in recommendation accuracy.
47%
uses AI daily
47% answered they interact with AI daily. AI is getting a part of users daily life.
78%
uses generative AI most frequently among AI services
Generative AI is one of the most frequently encountered AI services.
Dynamic preferences for music
Users' preferences are dynamic depending on moods and genres. Users can get disappointed by the music selected by online music platforms because they don't consider them.
Want to discover more diverse music
Users are open to exploring more diverse, new music that matches their preferences instead of repetitive playlists that are similar to the current listening habit.
Easier and quicker way to start listening to music
Users listen to music in diverse settings including driving, dancing, and so on. Users prefer fewer interactions to listen to their music.
Mood, artists and genres are the most important factors.
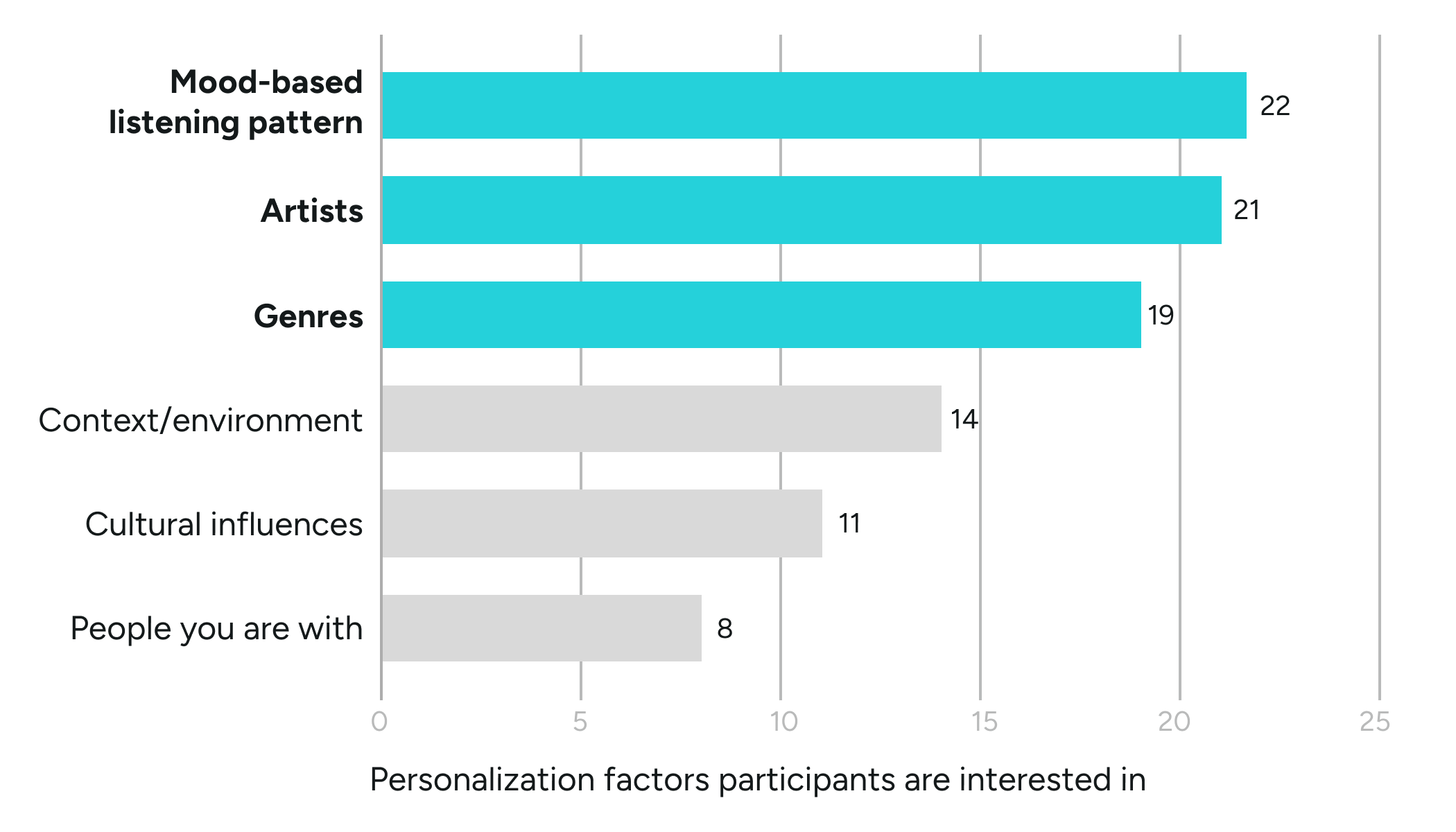
Amazon Music Chalenge Prompt
Given the current state of Amazon Music, how might AI enhance a customer’s experience with music and/or podcasts?
Design Process
We followed the design thinking process in this project.
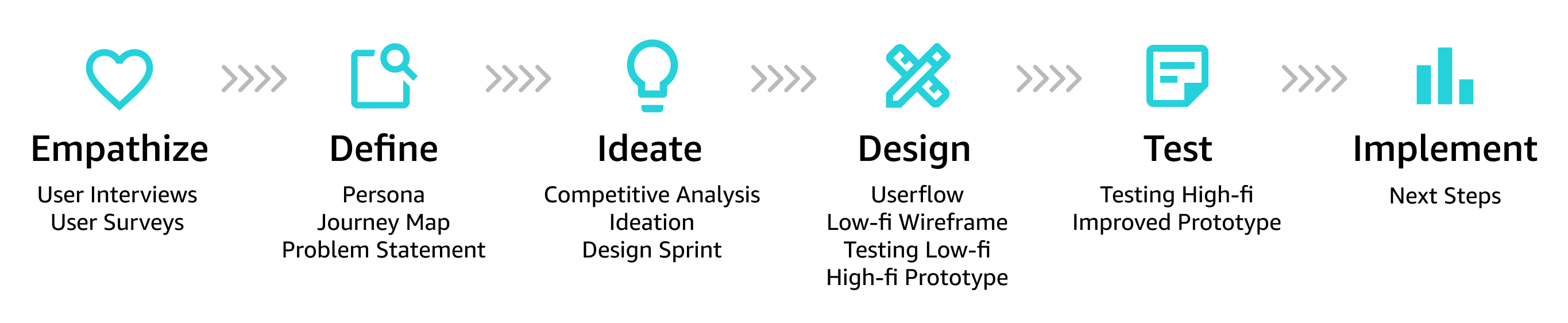
We conducted a competitive analysis of major music streaming products our interview participants use. As a result, we found opportunities in the ability to customize auto-generated playlists and control discovery levels directly.
Competitor Analysis on Personalization of Music Streaming Services
%20(1).png)
We held an in-person brainstorming session using the crazy8 method.
Set music preferences
Users can set their music preferences dynamically before they start listening to music. They can set the following options.
Discovery level (the ratio of new and familiar music)
Generate AI custom playlist
The generative AI creates a custom playlist based on users' preferences and generates the following.
Playlist that matches the preferences
Enjoy listening
Users can discover music that perfectly matches their moods and preferences. They can also perform the following actions.
We created the following low-fidelity wireframes. Then, we tested the low-fi wireframes with 10 participants in moderated sessions.
User Testing Findings
The labels are confusing
Difficult to associate “Mode” with the ratio of familiar/unfamiliar songs, and “Want more?” as the additional prompt input.
The country setting is unnecessary
Overall, users are satisfied with the setting categories but they think the country is not necessary because the country is a minor factor as a preference option and users can specify country preference as an additional prompt.
Mood options should be diverse and easy to select
Not only emotions but also other factors such as contexts influence users' moods, so we need more diverse options.
Based on the result of user testing, we created high-fidelity prototypes. We tested them again with 5 participants in moderated sessions. Here is the final design after that.
Low-fidelity Wireframes
We created low-fidelity wireframes.
We tested our high-fidelity wireframes with 5 participants in moderated sessions. Users were given a task to go through the prototype in this session and observed the process they doing the task.
Categorization within moods is unnecessary
In our initial prototype, we categorized the mood options. However, since mood is difficult to categorize, users prefer to have no category.
“Addition” label still requires clarity
Users mentioned the label “addition” is still confusing. They suggested giving users a hint to help users understand what they can use with the feature.
Improve the visual design of preference setting page
We got feedback to improve the visual design of addition input field to keep consistency. Also, distinguishing moods and genre design can help users understand they are two different categories of preference.
Research more about the strength and weakness of new technologies
Explore more intuitive methods to detect users' moods
Explore more intuitive UX writing
What I've learned is that problem-solving stands as the cornerstone of design and AI, and other technologies serve as tools to address these issues, not as a purpose. Although the use of AI was a requirement, we tried to focus on finding users' problems first before thinking about how to apply AI.
Furthermore, a key challenge of this project was conceiving a solution that effectively harnesses the strengths of AI technology. I learned understanding the strengths and weaknesses of new technologies is key.
Also, I've come to realize the complexity of detecting users' internal states, such as moods. We've decided to assist users in selecting moods through images, yet I recognize that this approach demands user effort to explore and select relevant options. I am eager to explore more intuitive methods for detecting emotions.
Lastly, I would like to further explore UX writing of labels because our solution still needs improvement to let users understand our new concept.